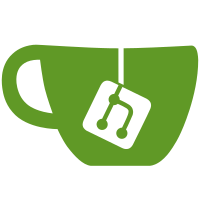
Questions for functionalities_test.py corrected some fixes Redo and implementation of everything path related now using pathlib.Path
241 lines
8.6 KiB
Python
241 lines
8.6 KiB
Python
from pathlib import Path
|
|
from tokenize import String
|
|
from typing import List, Dict
|
|
|
|
from tqdm import tqdm
|
|
import matplotlib.pyplot as plt
|
|
import matplotlib.patches as mpatches
|
|
import numpy as np
|
|
from sklearn.decomposition import PCA
|
|
import random
|
|
import string
|
|
|
|
|
|
def plot_output(output):
|
|
""" Plotting the values of the final output """
|
|
plt.figure()
|
|
plt.imshow(output)
|
|
plt.colorbar()
|
|
plt.show()
|
|
|
|
|
|
def plot_loss(loss_array, directory, batch_size=1):
|
|
""" Plotting the evolution of the loss function."""
|
|
|
|
fig = plt.figure()
|
|
fig.set_figheight(10)
|
|
fig.set_figwidth(12)
|
|
|
|
for i in range(len(loss_array)):
|
|
plt.plot(loss_array[i], label=f"Last loss value: {str(loss_array[i][len(loss_array[i])-1])}")
|
|
|
|
plt.legend()
|
|
plt.xlabel("Epochs")
|
|
plt.ylabel("Loss")
|
|
|
|
directory = Path(directory)
|
|
filename = "nets_loss_function.png"
|
|
file_path = directory / filename
|
|
plt.savefig(str(file_path))
|
|
|
|
plt.clf()
|
|
|
|
|
|
def bar_chart_fixpoints(fixpoint_counter: Dict, population_size: int, directory: String, learning_rate: float,
|
|
exp_details: String, source_check=None):
|
|
""" Plotting the number of fixpoints in a barchart. """
|
|
|
|
fig = plt.figure()
|
|
fig.set_figheight(10)
|
|
fig.set_figwidth(12)
|
|
|
|
legend_population_size = mpatches.Patch(color="white", label=f"No. of nets: {str(population_size)}")
|
|
learning_rate = mpatches.Patch(color="white", label=f"Learning rate: {str(learning_rate)}")
|
|
epochs = mpatches.Patch(color="white", label=f"{str(exp_details)}")
|
|
|
|
if source_check == "summary":
|
|
plt.legend(handles=[legend_population_size, learning_rate, epochs])
|
|
plt.ylabel("No. of nets/run")
|
|
plt.title("Summary: avg. amount of fixpoints/run")
|
|
else:
|
|
plt.legend(handles=[legend_population_size, learning_rate, epochs])
|
|
plt.ylabel("Number of networks")
|
|
plt.title("Fixpoint count")
|
|
|
|
plt.bar(range(len(fixpoint_counter)), list(fixpoint_counter.values()), align='center')
|
|
plt.xticks(range(len(fixpoint_counter)), list(fixpoint_counter.keys()))
|
|
|
|
directory = Path(directory)
|
|
filename = f"{str(population_size)}_nets_fixpoints_barchart.png"
|
|
filepath = directory / filename
|
|
plt.savefig(str(filepath))
|
|
|
|
plt.clf()
|
|
|
|
|
|
def plot_3d(matrices_weights_history, directory, population_size, z_axis_legend, exp_name="experiment", is_trained="",
|
|
batch_size=1):
|
|
""" Plotting the the weights of the nets in a 3d form using principal component analysis (PCA) """
|
|
|
|
fig = plt.figure()
|
|
fig.set_figheight(10)
|
|
fig.set_figwidth(12)
|
|
|
|
pca = PCA(n_components=2, whiten=True)
|
|
ax = plt.axes(projection='3d')
|
|
|
|
loop_matrices_weights_history = tqdm(range(len(matrices_weights_history)))
|
|
for i in loop_matrices_weights_history:
|
|
loop_matrices_weights_history.set_description("Plotting weights 3D PCA %s" % i)
|
|
|
|
weight_matrix, start_time = matrices_weights_history[i]
|
|
weight_matrix = np.array(weight_matrix)
|
|
n, x, y = weight_matrix.shape
|
|
weight_matrix = weight_matrix.reshape(n, x * y)
|
|
|
|
pca.fit(weight_matrix)
|
|
weight_matrix_pca = pca.transform(weight_matrix)
|
|
|
|
xdata, ydata = [], []
|
|
for j in range(len(weight_matrix_pca)):
|
|
xdata.append(weight_matrix_pca[j][0])
|
|
ydata.append(weight_matrix_pca[j][1])
|
|
zdata = np.arange(start_time, len(ydata)*batch_size+start_time, batch_size).tolist()
|
|
|
|
ax.plot3D(xdata, ydata, zdata)
|
|
ax.scatter(np.array(xdata), np.array(ydata), np.array(zdata), s=7)
|
|
|
|
steps = mpatches.Patch(color="white", label=f"{z_axis_legend}: {len(matrices_weights_history)} steps")
|
|
population_size = mpatches.Patch(color="white", label=f"Population: {population_size} networks")
|
|
|
|
if z_axis_legend == "Self-application":
|
|
trained = mpatches.Patch(color="white", label=f"Trained: true") if is_trained == "_trained" else mpatches.Patch(color="white", label=f"Trained: false")
|
|
ax.legend(handles=[steps, population_size, trained])
|
|
else:
|
|
ax.legend(handles=[steps, population_size])
|
|
|
|
ax.set_title(f"PCA Weights history")
|
|
ax.set_xlabel("PCA X")
|
|
ax.set_ylabel("PCA Y")
|
|
ax.set_zlabel(f"Epochs")
|
|
|
|
# FIXME: Replace this kind of operation with pathlib.Path() object interactions
|
|
directory = Path(directory)
|
|
directory.mkdir(parents=True, exist_ok=True)
|
|
filename = f"{exp_name}{is_trained}.png"
|
|
filepath = directory / filename
|
|
if filepath.exists():
|
|
letters = string.ascii_lowercase
|
|
random_letters = ''.join(random.choice(letters) for _ in range(5))
|
|
plt.savefig(f"{filepath.stem}_{random_letters}.png")
|
|
else:
|
|
plt.savefig(str(filepath))
|
|
|
|
plt.show()
|
|
|
|
|
|
def plot_3d_self_train(nets_array: List, exp_name: String, directory: String, batch_size: int):
|
|
""" Plotting the evolution of the weights in a 3D space when doing self training. """
|
|
|
|
matrices_weights_history = []
|
|
|
|
loop_nets_array = tqdm(range(len(nets_array)))
|
|
for i in loop_nets_array:
|
|
loop_nets_array.set_description("Creating ST weights history %s" % i)
|
|
|
|
matrices_weights_history.append( (nets_array[i].s_train_weights_history, nets_array[i].start_time) )
|
|
|
|
z_axis_legend = "epochs"
|
|
|
|
return plot_3d(matrices_weights_history, directory, len(nets_array), z_axis_legend, exp_name, "", batch_size)
|
|
|
|
|
|
def plot_3d_self_application(nets_array: List, exp_name: String, directory_name: String, batch_size: int) -> None:
|
|
""" Plotting the evolution of the weights in a 3D space when doing self application. """
|
|
|
|
matrices_weights_history = []
|
|
|
|
loop_nets_array = tqdm(range(len(nets_array)))
|
|
for i in loop_nets_array:
|
|
loop_nets_array.set_description("Creating SA weights history %s" % i)
|
|
|
|
matrices_weights_history.append( (nets_array[i].s_application_weights_history, nets_array[i].start_time) )
|
|
|
|
if nets_array[i].trained:
|
|
is_trained = "_trained"
|
|
else:
|
|
is_trained = "_not_trained"
|
|
|
|
# Fixme: Are the both following lines on the correct intendation? -> Value of "is_trained" changes multiple times!
|
|
z_axis_legend = "epochs"
|
|
plot_3d(matrices_weights_history, directory_name, len(nets_array), z_axis_legend, exp_name, is_trained, batch_size)
|
|
|
|
|
|
def plot_3d_soup(nets_list, exp_name, directory):
|
|
""" Plotting the evolution of the weights in a 3D space for the soup environment. """
|
|
|
|
# This batch size is not relevant for soups. To not affect the number of epochs shown in the 3D plot,
|
|
# will send forward the number "1" for batch size with the variable <irrelevant_batch_size>.
|
|
irrelevant_batch_size = 1
|
|
|
|
plot_3d_self_train(nets_list, exp_name, directory, irrelevant_batch_size)
|
|
|
|
|
|
def line_chart_fixpoints(fixpoint_counters_history: list, epochs: int, ST_steps_between_SA: int,
|
|
SA_steps, directory: String, population_size: int):
|
|
""" Plotting the percentage of fixpoints after each iteration of SA & ST steps. """
|
|
|
|
fig = plt.figure()
|
|
fig.set_figheight(10)
|
|
fig.set_figwidth(12)
|
|
|
|
ST_steps_per_SA = np.arange(0, ST_steps_between_SA * epochs, ST_steps_between_SA).tolist()
|
|
|
|
legend_population_size = mpatches.Patch(color="white", label=f"No. of nets: {str(population_size)}")
|
|
legend_SA_steps = mpatches.Patch(color="white", label=f"SA_steps: {str(SA_steps)}")
|
|
legend_SA_and_ST_runs = mpatches.Patch(color="white", label=f"SA_and_ST_runs: {str(epochs)}")
|
|
legend_ST_steps_between_SA = mpatches.Patch(color="white", label=f"ST_steps_between_SA: {str(ST_steps_between_SA)}")
|
|
|
|
plt.legend(handles=[legend_population_size, legend_SA_and_ST_runs, legend_SA_steps, legend_ST_steps_between_SA])
|
|
plt.xlabel("Epochs")
|
|
plt.ylabel("Percentage")
|
|
plt.title("Percentage of fixpoints")
|
|
|
|
plt.plot(ST_steps_per_SA, fixpoint_counters_history, color="green", marker="o")
|
|
|
|
directory = Path(directory)
|
|
filename = f"{str(population_size)}_nets_fixpoints_linechart.png"
|
|
filepath = directory / filename
|
|
plt.savefig(str(filepath))
|
|
|
|
plt.clf()
|
|
|
|
|
|
def box_plot(data, directory, population_size):
|
|
fig, axs = plt.subplots(nrows=1, ncols=2, figsize=(10, 7))
|
|
|
|
# ax = fig.add_axes([0, 0, 1, 1])
|
|
plt.title("Fixpoint variation")
|
|
plt.xlabel("Amount of noise")
|
|
plt.ylabel("Steps")
|
|
|
|
# data = numpy.array(data)
|
|
# ax.boxplot(data)
|
|
axs[1].boxplot(data)
|
|
axs[1].set_title('Box plot')
|
|
|
|
directory = Path(directory)
|
|
filename = f"{str(population_size)}_nets_fixpoints_barchart.png"
|
|
filepath = directory / filename
|
|
|
|
plt.savefig(str(filepath))
|
|
plt.clf()
|
|
|
|
|
|
def write_file(text, directory):
|
|
directory = Path(directory)
|
|
filepath = directory / 'experiment.txt'
|
|
with filepath.open('w+') as f:
|
|
f.write(text)
|
|
f.close()
|